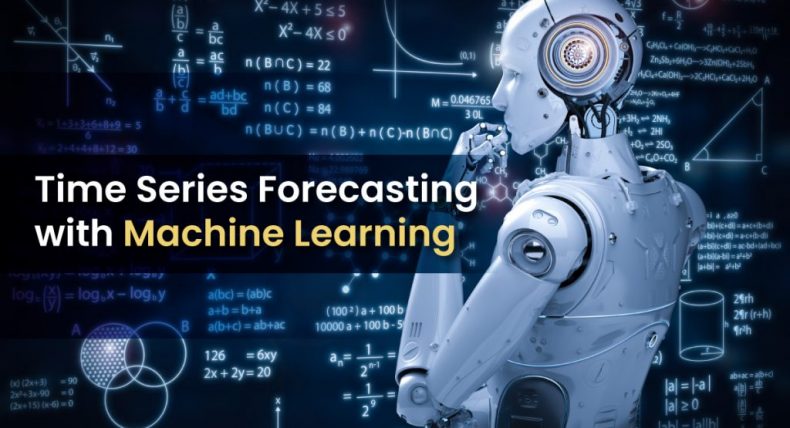
Four Guiding Principles for Success in Time Series Forecasting
1. Understand the Different Time Series Patterns
Time series includes trend cycles and seasonality. Unfortunately, many confuse seasonal behavior with cyclic behavior. To avoid confusion, let’s understand what they are:
- Trend: An increase or decrease in data over a period of time is called a trend. They could be deterministic, which provides an underlying rationale, or stochastic, which is a random feature of time series.
- Seasonal: Oftentimes, seasonality is of a fixed and known frequency. When a time series is affected by seasonal factors like the time of the year or the day of the week, a seasonal pattern occurs.
- Cyclic: When a data exhibit fluctuates, a cycle occurs. But unlike seasonal, it is not of a fixed frequency.
2. Use Features Carefully
It is important to use features carefully, especially when their future real values are unclear. However, if the features are predictable or have patterns you will be able to build a forecast model based on them. Using predicted values as features is risky as it can cause substantial errors and provide a biased result. Properties of a time series and time-related features that can be calculated could be added to time series models. Mistakes in handle features could easily get compounded resulting in extremely skewed results, so extreme caution is in order.
3. Be Prepared to Handle Smaller Time Series
Don’t be quick to dismiss smaller time series as a drawback. All time-related datasets are useful in time series forecasting. A smaller dataset wouldn’t require external memory for your computer, which makes it easier to analyze the entire dataset and make plots that could be analyzed graphically.
4. Choose The Right Resolution
Having a clear idea of the objectives of your analysis will help yield better results. It will reduce the risk of propagating the error to the total. An unbiased model’s residuals would either be zero or close to zero. A white noise series is expected to have all autocorrelations close to zero. In other words, choosing the right resolution will also eliminate noisy data that makes modeling difficult.
Types of Time Series Data and Forecasts
Times series basically deals with three types of data – time-series data, cross-sectional data, and pooled data, which is a combination of time series data and cross-sectional data. Large amounts of data give you the opportunity for exploratory data analysis, model fidelity and model testing and tuning. The question you could ask yourself is, how much data is available and how much data am I able to collect?
There are different types of forecasting that could be applied depending on the time horizon. They are near-future, medium-future and long-term future predictions. Think carefully about which time horizon prediction you need.
Organizations should be able to decide which forecast works best for their firm. A rolling forecast will re-forecast the next twelve months, whereas the traditional, or a static annual forecast creates new forecasts towards the end of the year. Think about whether you want your forecasts updated regularly or you need a more static approach.
By allowing you to harness down-sampling and up-sampling data, the concept of temporal hierarchies can mitigate modeling uncertainty. It is important to ask yourself, what temporal frequencies require forecasts?
Keep Up With Time
As businesses grow more dynamic, forecasting will get increasingly harder because of the increasing amount of data needed to build the Time Series Forecasting model. Still, implementing the principles outlined in this blog will help your organization be better equipped for success.